Revolutionizing Tech: Edge AI & TinyML Explained
- Baveling
- Mar 26
- 9 min read
In an era where speed and efficiency are paramount, the convergence of artificial intelligence with real-time data processing is not just advantageous—it's essential. Enter Edge AI and Tiny Machine Learning (TinyML), two revolutionary technologies that stand at the forefront of this transformative wave. As the digital landscape evolves, these technologies are not merely enhancing capabilities; they are redefining the possible.
Edge AI brings the power of artificial intelligence to the edge of the network, directly onto the devices we use every day. From smartphones to smart home devices, Edge AI processes data locally, minimizing latency and conserving bandwidth. Complementing this, TinyML—a subset of machine learning—operates on even the smallest microcontrollers, enabling AI applications where conventional technologies cannot tread due to power and space constraints.
This article delves into the intricate dance of Edge AI and TinyML, exploring how they are not just shaping the future of technology but are actively carving out new realms of possibilities in real-time applications. Join us as we uncover the layers of Edge AI and TinyML, their impacts, their challenges, and the untapped potential they hold in revolutionizing industries and day-to-day life. Whether you're a tech enthusiast, a professional in the tech industry, or simply curious about the future of AI, this exploration will illuminate the significance and transformative power of these cutting-edge technologies.
Understanding Edge AI
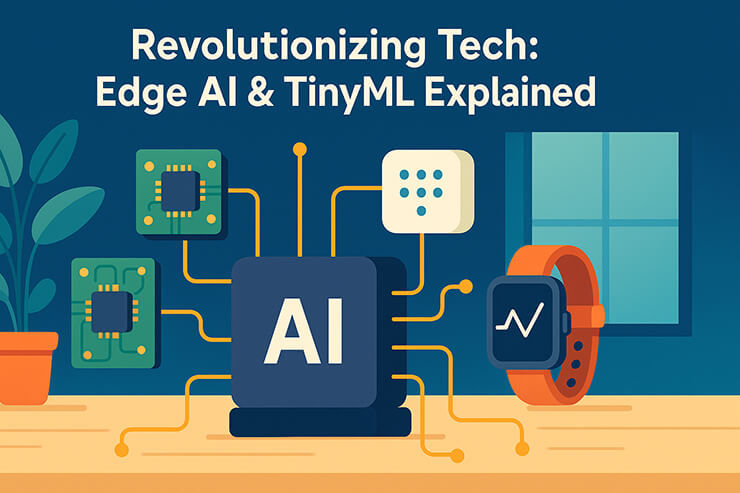
What is Edge AI?
Edge AI refers to the deployment of artificial intelligence algorithms directly on a local device, operating at the data source rather than relying on cloud-based services. This means that AI processing happens right where data is generated—be it a mobile phone, an industrial machine, or an autonomous vehicle. This approach allows devices to perform real-time data processing, react instantly to changes, and operate independently without needing constant communication with a central server.
Key Differences Between Edge AI and Cloud-Based AI
While traditional AI systems rely heavily on cloud computing, Edge AI shifts the processing workload to the edge of the network. Here are some crucial distinctions:
Latency: Edge AI significantly reduces the delay in data processing by eliminating the need to send data back and forth to the cloud, providing almost instantaneous responses.
Privacy and Security: By processing data locally, Edge AI minimizes the risk of data breaches and exposure during transmission, offering a more secure form of data handling that is particularly crucial in sensitive environments like healthcare or finance.
Bandwidth and Cost Efficiency: Local data processing reduces the dependency on internet bandwidth, which can be costly and limited in some regions. This not only saves on operational costs but also ensures functionality in areas with poor connectivity.
Benefits of Edge AI
The implementation of Edge AI brings several benefits that are transforming industries:
Enhanced Performance: Devices can operate in real-time with reduced latency, improving user experience and device efficiency.
Operational Reliability: Devices can continue to operate effectively even when offline or in unstable network conditions.
Scalability: As AI capabilities grow, scaling Edge AI solutions becomes more feasible without the proportional increase in bandwidth usage or cloud storage costs.
Edge AI is not just a technological upgrade but a strategic advantage that is rapidly becoming a staple in industries ranging from telecommunications to healthcare, from retail to automotive. As we continue to generate more data, the importance of processing it at the source is becoming increasingly apparent, paving the way for a smarter, more connected world.
Exploring TinyML
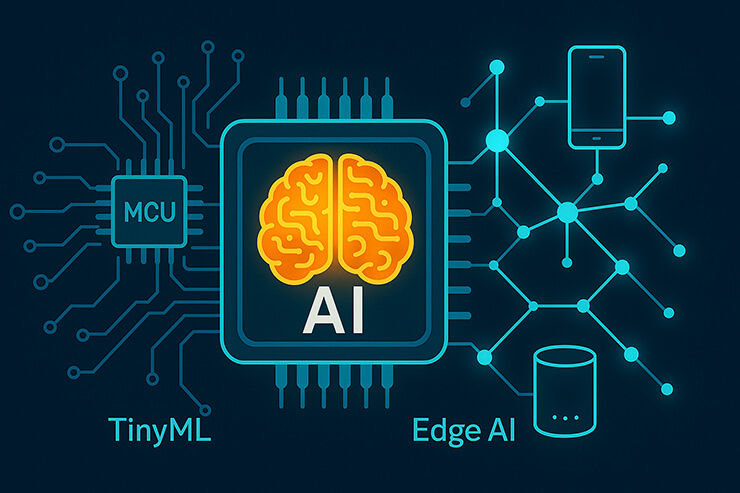
Introduction to TinyML
Tiny Machine Learning (TinyML) is at the cutting edge of minimizing machine learning systems to fit on tiny hardware. It involves deploying lightweight machine learning models on low-power devices such as microcontrollers. These are embedded systems that can operate on power equivalent to a standard watch battery, and they can be found in billions of everyday devices—from wearables to agricultural sensors.
Role of TinyML in Enhancing Edge AI Capabilities
TinyML is pivotal in pushing the boundary of where and how AI can be implemented, especially in scenarios where power consumption, space, and processing capabilities are limited. Here’s how TinyML is enhancing Edge AI capabilities:
Extended Reach: By enabling AI on microdevices, TinyML extends the reach of intelligent solutions to virtually any device. This ubiquity of AI facilitates smarter consumer products, more efficient industrial machines, and innovative solutions in areas previously untouched by high-tech due to power and size constraints.
Increased Efficiency: TinyML models are specifically designed to be extremely efficient. They require fewer computational resources and less power, which not only preserves the device's battery life but also reduces costs associated with energy use and hardware maintenance.
Real-Time Decision Making: With TinyML, devices can process data and make decisions locally, without the latency associated with sending data to a distant server. This capability is crucial for applications requiring immediate response, such as emergency health alerts or real-time environmental monitoring.
Examples of TinyML Applications
The potential applications of TinyML are vast and varied. Here are a few real-world examples where TinyML is making an impact:
Healthcare Monitoring: Wearable devices that monitor health metrics like heart rate and sleep patterns use TinyML to provide real-time insights and alerts.
Agricultural Optimization: Sensors deployed in agricultural fields that predict soil conditions and crop health, enabling farmers to make informed decisions without the need for extensive manual monitoring.
Smart Homes: TinyML is used in smart home devices such as thermostats and lighting systems, which learn user preferences and adjust settings automatically to optimize comfort and energy use.
Challenges Facing TinyML
Despite its significant potential, TinyML faces challenges such as:
Model Accuracy: Due to the limited computing power, TinyML models may not always achieve the same accuracy as their larger counterparts.
Development Complexity: Designing effective models that run on such limited resources requires specialized knowledge and tools, which can be a barrier for widespread adoption.
Key Technologies Behind Edge AI and TinyML

Hardware Essentials for Edge AI
The implementation of Edge AI requires robust hardware capable of supporting AI computations locally. Some key hardware components include:
GPUs and Specialized Accelerators: Many edge devices incorporate GPUs for faster processing speeds. Additionally, specialized accelerators like Google's Edge TPU are designed to efficiently run AI models at the edge, significantly boosting performance.
Microcontrollers and Sensors: TinyML thrives on microcontrollers—small, low-power computing devices that can perform AI tasks without the need for extensive power. These are often coupled with sensors that collect data from the environment, forming the foundation of many IoT (Internet of Things) applications.
FPGAs (Field Programmable Gate Arrays): These devices can be reprogrammed to suit specific needs and are increasingly used in Edge AI for their flexibility and efficiency.
Software Frameworks and Tools
To build and deploy Edge AI and TinyML models, developers rely on a range of software frameworks and tools:
TensorFlow Lite: This lightweight version of TensorFlow is specifically designed for mobile and edge devices. It allows developers to convert full-sized models into versions suitable for low-power and low-latency environments.
PyTorch Mobile: Similar to TensorFlow Lite, PyTorch Mobile is optimized for mobile and embedded devices, facilitating the integration of PyTorch models into Edge AI applications.
Edge Impulse: Focusing on TinyML, Edge Impulse provides an end-to-end platform that enables the rapid development and deployment of machine learning models on edge devices.
Integration of AI with IoT
The marriage of AI with IoT has opened up new vistas for Edge AI and TinyML. By integrating intelligent algorithms directly into IoT devices, businesses and consumers can leverage:
Automated Decision-Making: Devices can analyze data and make decisions independently without human intervention.
Predictive Maintenance: In industrial settings, AI-enabled sensors can predict equipment failures before they occur, saving time and money.
Enhanced User Experience: From smart homes to wearables, AI-driven IoT devices adapt to user behaviors and preferences, providing a more personalized experience.
Challenges and Considerations
While the integration of these technologies promises substantial benefits, several challenges need to be navigated:
Security Concerns: As more devices become smarter and more connected, they also become potential targets for cyber threats.
Interoperability Issues: Ensuring that different devices and platforms can work together seamlessly remains a significant challenge.
Development and Deployment Costs: While hardware costs are decreasing, the cost of developing and maintaining sophisticated systems can be prohibitive for some organizations.
Advantages of Edge AI and TinyML
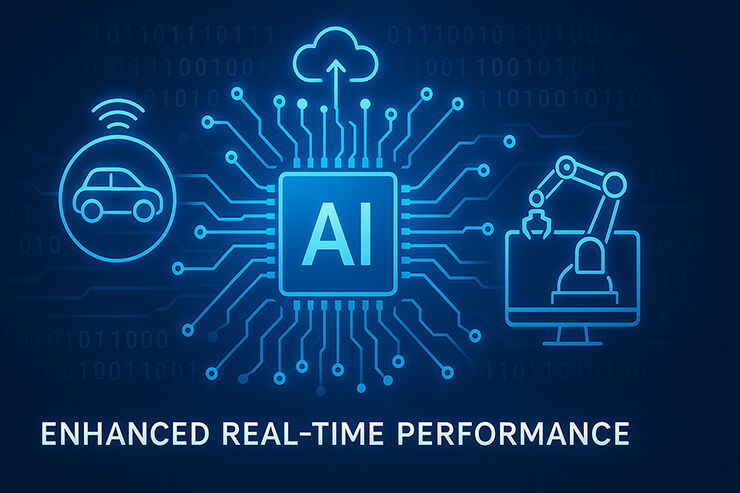
Enhanced Real-Time Performance
One of the most significant benefits of Edge AI and TinyML is their ability to enhance real-time performance across various devices and applications. By processing data locally, these technologies enable devices to react instantly to inputs without the latency associated with data transmission to and from the cloud. This is critical in applications such as autonomous vehicles, where split-second decisions can be a matter of safety, or in manufacturing, where real-time adjustments are crucial for maintaining quality and efficiency.
Increased Privacy and Data Security
With Edge AI, data is processed directly on the device, significantly reducing the risks associated with data breaches during transmission. This local processing means sensitive information, such as personal health data or financial information, does not need to be sent to the cloud, thereby enhancing privacy and security—a paramount concern in today’s digital age.
Operational Reliability and Independence
Edge AI enables devices to operate independently of the cloud, which is especially valuable in environments with unstable or limited connectivity. For instance, in remote areas or during network outages, Edge AI devices can continue functioning, ensuring continuous operations in critical sectors like healthcare and emergency services.
Cost-Effective and Energy Efficient
By minimizing the need for continuous data transmission to the cloud, Edge AI and TinyML reduce bandwidth usage and associated costs. Furthermore, TinyML’s ability to run on low-power devices leads to significant energy savings, making it ideal for scaling up deployments in cost-sensitive environments.
Scalability Across Industries
The scalability of Edge AI and TinyML technologies allows them to be deployed across a broad spectrum of industries. They are not limited to high-tech sectors but can be integrated into everyday consumer products, industrial equipment, and even across the agricultural sector, demonstrating a versatile range of applications from smart thermostats to precision farming tools.
Challenges and Limitations
Despite their numerous advantages, Edge AI and TinyML face several challenges that must be considered:
Resource Constraints: Operating on the edge often means working within the constraints of limited computational power and memory, which can impact the complexity and capability of AI models.
Maintenance and Updates: Managing and updating AI models across thousands of distributed devices can pose logistical challenges.
Development Complexity: Building effective AI solutions that operate under these constraints requires specialized knowledge and resources, which can limit accessibility and widespread adoption.
The Future of Edge AI and TinyML
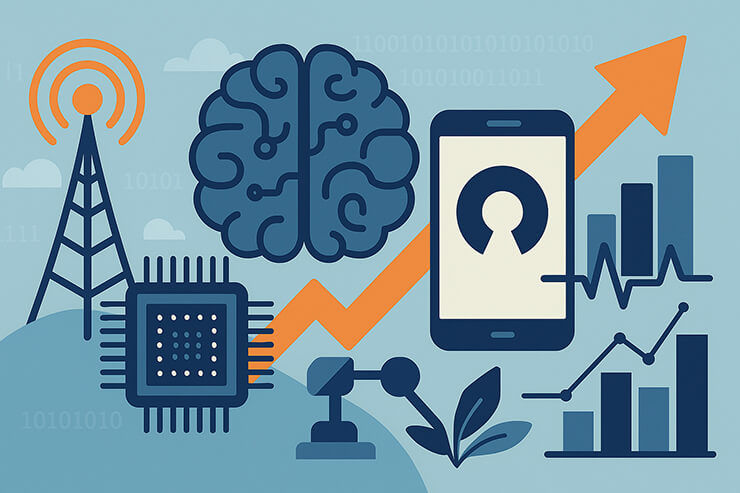
Emerging Trends and Developments
As technology continues to advance, Edge AI and TinyML are expected to play even more critical roles in a wide array of sectors. Here are some key trends to watch:
Greater Integration with 5G Technology: The rollout of 5G networks will enhance the capabilities of Edge AI by reducing latency even further and increasing the speed and volume of data that can be processed at the edge. This will be particularly transformative for applications requiring real-time responses, such as autonomous driving and advanced robotics.
Advancements in Hardware Efficiency: Continued improvements in microprocessor and sensor technology will allow for even smaller, more power-efficient devices capable of performing complex computations. This will expand the potential applications of TinyML to virtually every electronic device.
AI Democratization through Open Source Projects: The growth of open-source frameworks and tools will lower barriers to entry for developers wanting to implement Edge AI and TinyML. This democratization of technology will likely spur innovation across numerous industries and geographic regions.
Cross-Domain Synergies: As more industries adopt Edge AI and TinyML, synergies between different applications will emerge. For example, techniques refined in the healthcare sector might find applications in industrial monitoring, leading to cross-pollination of ideas and innovations.
Ethical AI at the Edge: With increasing awareness of the ethical implications of AI, there will be a stronger focus on developing responsible AI algorithms that are transparent and fair. This trend will be critical in maintaining public trust as AI becomes more embedded in everyday life.
Potential Impact on Industries
The influence of Edge AI and TinyML is set to expand across multiple domains:
Healthcare: Enhanced diagnostic tools and real-time patient monitoring systems will improve outcomes and accessibility to medical care, especially in remote areas.
Automotive: Smarter, safer autonomous vehicles equipped with Edge AI will become more reliable, paving the way for widespread adoption.
Manufacturing: Increased automation and improved efficiency on production lines will reduce costs and enhance product quality.
Consumer Electronics: Devices will become more personalized, adjusting in real-time to user behaviors and preferences, enhancing the user experience and device utility.
Challenges Ahead
While the future is promising, significant challenges remain, such as ensuring the security of edge devices, managing the proliferation of devices, and developing sustainable models that balance performance with energy use. Addressing these challenges will be crucial for the successful deployment of Edge AI and TinyML technologies on a global scale.
Conclusion: Harnessing the Power of Edge AI and TinyML for a Smarter Future
As we have explored throughout this article, Edge AI and TinyML represent more than just technological advancements; they are pivotal forces driving the evolution of intelligent systems across the globe. These technologies empower devices to process data locally, make real-time decisions, and operate with unprecedented efficiency and autonomy. The implications for industries ranging from healthcare to automotive, and from agriculture to consumer electronics, are profound and far-reaching.
Recap of Key Points
Edge AI enhances real-time data processing, reduces latency, and ensures data privacy and security by localizing operations.
TinyML extends the capabilities of machine learning to microcontrollers and small devices, enabling AI applications in environments where power and space are limited.
Together, these technologies foster innovative solutions that improve efficiency, enhance user experiences, and drive economic and environmental benefits.
The Road Ahead
Looking forward, the continued integration of Edge AI and TinyML with emerging technologies like 5G, advanced sensors, and more sophisticated machine learning models promises to unlock even greater potential. As hardware becomes more capable and software more intelligent, the boundaries of what's possible will continue to expand.
However, to fully capitalize on these benefits, it is crucial to address the challenges related to security, interoperability, and ethical considerations. Developers, policymakers, and stakeholders must collaborate to create robust frameworks that ensure these technologies are used responsibly and benefit society as a whole.