In the rapidly evolving world of artificial intelligence, the quest for models that mimic human reasoning and decision-making continues to push technological boundaries. Enter Neurosymbolic AI, a cutting-edge field that merges the intuitive processing power of neural networks with the logical precision of symbolic artificial intelligence. This hybrid approach promises to revolutionize how machines understand and interact with the world around them.
At its core, Neurosymbolic AI aims to create systems that are not only adept at handling large volumes of data but are also capable of understanding and manipulating symbolic information. This integration marks a significant step forward from traditional AI methods, which typically excel in pattern recognition but struggle with complex reasoning tasks. By bridging this gap, Neurosymbolic AI is poised to transform a myriad of sectors, including natural language processing, robotics, and complex decision-making systems.
In this article, we will explore the fundamentals of Neurosymbolic AI, delve into its unique workings, and uncover the profound impacts it could have across various industries. Join us as we unravel the potential of Neurosymbolic AI to bring us closer to developing machines that can think, reason, and understand like humans.
What is Neurosymbolic AI?
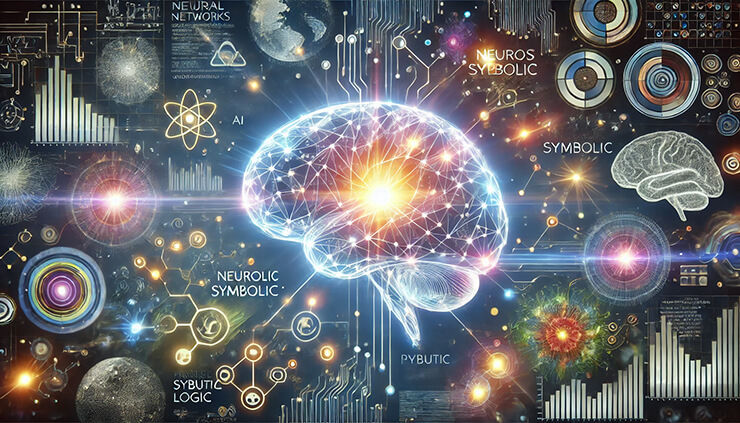
Neurosymbolic AI represents a transformative approach in the field of artificial intelligence that synthesizes two distinct methodologies: the dynamic learning capabilities of neural networks and the rule-based processing of symbolic AI. This combination aims to leverage the strengths of each to overcome their individual limitations, thereby enabling more sophisticated, versatile AI systems.
Understanding the Components
Neural Networks: At one end of the spectrum, neural networks are adept at processing and making sense of vast amounts of unstructured data. They learn from examples and can recognize patterns and nuances that are not explicitly programmed. This makes them invaluable for tasks like image and speech recognition.
Symbolic AI: On the other end, symbolic AI operates on clear, definable rules and structured data. It excels in tasks that require logical reasoning and decision-making based on explicit knowledge bases. Unlike neural networks, symbolic AI does not learn from data but follows predefined logic to process information.
Integration of Neural Networks and Symbolic AI
The integration of these two AI forms aims to create a system where:
Neural networks can provide an understanding of raw, unstructured data.
Symbolic AI can structure this understanding into a form that is logically coherent and usable in decision-making processes.
This fusion enables machines to not only 'perceive' the world but also to 'understand' and 'reason' about it in a way that mimics human cognitive processes.
Why Neurosymbolic AI Matters
The significance of Neurosymbolic AI lies in its potential to:
Bridge the Semantic Gap: It can translate vague, abstract data from neural networks into precise, actionable information through symbolic AI.
Enhanced Reasoning: By combining intuition-like pattern recognition with rule-based processing, it enhances the machine's ability to reason from general principles to specific cases.
Robust Decision-Making: It supports more reliable decision-making by ensuring that the AI’s actions are both data-informed and rule-compliant, reducing errors that arise from data biases or logical inconsistencies.
This integration marks a pivotal advance in AI development, pushing us closer to creating machines that can engage with complex, real-world problems in a manner that is both intelligent and understandable.
How Does Neurosymbolic AI Work?
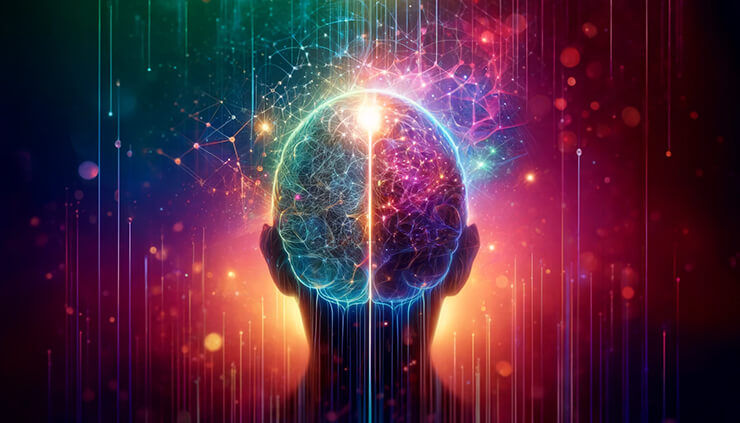
To appreciate the functionality of Neurosymbolic AI, it is essential to understand how it blends the intuitive data processing of neural networks with the structured reasoning capabilities of symbolic AI. This synergy not only enhances the effectiveness of AI applications but also introduces a new dimension of cognitive abilities in machines.
Step-by-Step Breakdown of the Neurosymbolic AI Process
Data Input and Initial Processing: Data enters the system, typically unstructured and vast—ranging from images and text to sensor data. Neural networks initially process this raw data, extracting features and patterns essential for further analysis.
Pattern Recognition and Preliminary Learning: The neural network segment of the system identifies patterns and learns from them, creating a preliminary understanding of the data. This stage is crucial for tasks like recognizing faces in images or sentiments in text.
Symbolic Transformation: The extracted features and recognized patterns are then transformed into symbolic representations. This conversion involves categorizing the neural network’s outputs into discrete, understandable symbols or concepts that can be manipulated logically.
Rule-Based Reasoning: Once in symbolic form, these elements are processed using the rules and logic predefined in the symbolic AI system. This stage allows the system to perform logical reasoning, make decisions, and even generate new hypotheses or conclusions based on the rules applied to the symbols.
Feedback and Refinement: The outcomes of the symbolic reasoning can feedback into the neural network, helping refine its learning process. This feedback loop can lead to progressively more accurate interpretations and decisions, as the neural network adjusts based on the logical outputs of the symbolic AI.
Visual Aids and Examples
Diagrams: Using flowcharts or diagrams can effectively illustrate the flow from neural network processing to symbolic reasoning, helping visualize the integration.
Case Studies: Including practical examples, such as a Neurosymbolic AI system used in a healthcare setting for diagnosing diseases from symptoms and medical images, can clarify how these processes work in real applications.
Algorithms at Play
Federated Averaging (FedAvg) and other algorithms may be employed in the neural network phases to optimize data learning.
Logic Programming: In the symbolic phase, techniques like Prolog or Datalog might be used for structuring and utilizing the symbolic data effectively.
By combining the deep learning capabilities of neural networks with the structured logical reasoning of symbolic AI, Neurosymbolic AI not only amplifies the potential applications of artificial intelligence but also moves closer to replicating a more human-like understanding and reasoning process in machines.
Key Benefits of Neurosymbolic AI
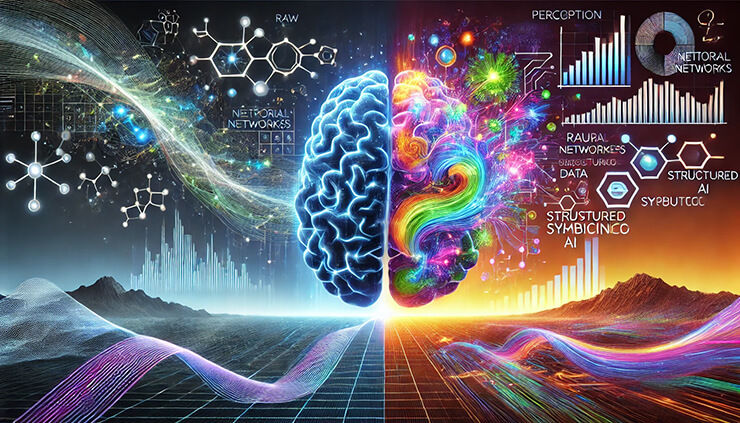
Neurosymbolic AI offers several transformative benefits that address the limitations of purely neural or symbolic approaches in artificial intelligence. This hybrid methodology enhances not only the scope of AI applications but also their efficiency and reliability.
Enhanced Reasoning and Decision Making
Neurosymbolic AI excels in combining the intuitive pattern recognition capabilities of neural networks with the logical precision of symbolic AI, resulting in superior reasoning and decision-making processes. This integration allows AI systems to not only recognize complex patterns but also to understand the implications of these patterns, enabling them to make informed decisions based on a deeper understanding of the context.
Improved Data Efficiency
Unlike traditional neural network approaches that require vast amounts of data to train effectively, Neurosymbolic AI can achieve significant learning with less data. By incorporating symbolic reasoning, these systems can utilize prior knowledge and logical rules, thereby reducing the dependency on large datasets and speeding up the training process.
Robust Generalization
Neurosymbolic AI systems are better equipped to generalize from limited examples. By understanding the underlying principles that govern the data, these systems can apply learned knowledge to new, unseen situations much more effectively than traditional AI. This is especially beneficial in fields where data can be scarce or expensive to obtain.
Transparency and Interpretability
One of the critical challenges in AI, particularly with deep learning models, is the lack of transparency and interpretability. Neurosymbolic AI addresses this by integrating symbolic AI, which operates based on understandable and explainable rules. This makes the decision-making process of AI systems more transparent and easier to audit, a crucial factor in sectors like healthcare and finance where explainability is mandatory.
Adaptability and Flexibility
Neurosymbolic AI systems are inherently more adaptable and flexible. They can quickly assimilate new rules and knowledge into their framework without needing extensive retraining. This ability to dynamically update and modify their knowledge base and rules makes them particularly suited for applications in rapidly changing environments.
Error Reduction
By logically reasoning about their actions and decisions, Neurosymbolic AI systems can reduce the error rates inherent in purely data-driven AI models. This error reduction is critical in high-stakes areas such as medical diagnostics, autonomous driving, and financial forecasting, where mistakes can have significant consequences.
Applications of Neurosymbolic AI

Neurosymbolic AI's unique capabilities open up a wealth of applications across various sectors. These applications not only demonstrate the practical benefits of integrating neural networks with symbolic AI but also highlight the transformative impact this technology can have on our everyday lives and decision-making processes.
Natural Language Processing (NLP)
Advanced Understanding and Generation: Neurosymbolic AI enhances NLP systems, enabling them to understand and generate human-like text with greater context and accuracy. This is crucial for applications such as virtual assistants, automated customer support, and real-time translation services.
Semantic Analysis: Beyond basic parsing of text, Neurosymbolic AI can infer deeper meanings, sentiments, and intentions from written or spoken language, improving interaction quality and effectiveness.
Robotics
Context-Aware Robotics: In robotics, Neurosymbolic AI helps machines to interpret complex environments and make informed decisions based on both sensory data and predefined rules. This is particularly useful in scenarios requiring interaction with humans or navigation in dynamic or unpredictable settings.
Enhanced Problem Solving: Robots equipped with Neurosymbolic AI can solve problems more effectively by applying logical reasoning to sensory inputs, which is vital for tasks like assembly in manufacturing or service delivery in homes and offices.
Complex Decision-Making Systems
Healthcare: In the healthcare sector, Neurosymbolic AI can be used to diagnose diseases more accurately by combining medical imaging data with clinical knowledge. This approach helps in identifying patterns that human experts may overlook and applying medical rules to ensure precise diagnostics.
Finance: Financial institutions can leverage Neurosymbolic AI for risk assessment, fraud detection, and investment decision-making by integrating quantitative data analysis with regulatory and economic principles.
Legal and Compliance: Automating compliance checks and legal assessments by understanding and applying regulations and legal precedents in data-driven contexts, such as contract analysis or regulatory reporting.
Educational Technologies
Personalized Learning: Neurosymbolic AI can tailor educational content to individual students’ learning styles and progress by interpreting their interaction data and aligning it with pedagogical rules.
Intelligent Tutoring Systems: These systems can provide real-time feedback and assistance by understanding complex student queries and generating instructive responses.
Gaming and Entertainment
Interactive Gameplay: Game development can benefit from Neurosymbolic AI by creating more engaging and responsive AI characters that react intelligently to player actions based on a mix of scripted rules and learned behaviors.
Dynamic Content Generation: In entertainment, Neurosymbolic AI can dynamically generate content that adapts to user preferences and interactions, enhancing personalized experiences.
Challenges in Neurosymbolic AI
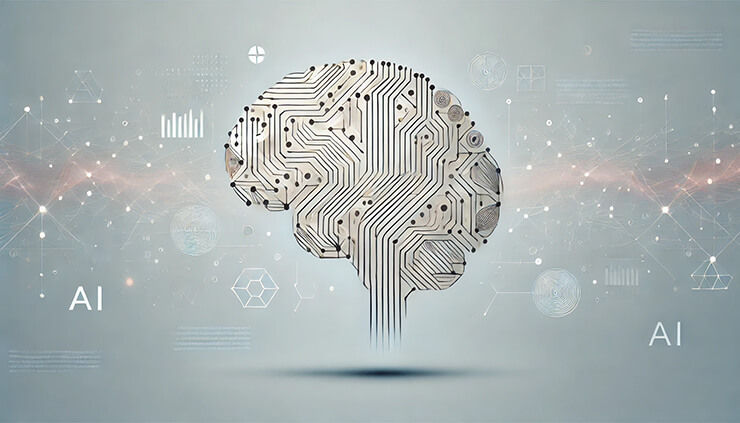
Despite its transformative potential, Neurosymbolic AI faces several challenges that can hinder its development and widespread adoption. Addressing these challenges is crucial for realizing the full potential of this innovative technology.
Integration Complexity
Combining Disparate Systems: Integrating neural networks with symbolic AI involves merging fundamentally different computational paradigms, which can be technically challenging. Ensuring seamless interaction between data-driven and rule-based components requires sophisticated architectural and algorithmic innovations.
Balancing Flexibility and Rigidity: Finding the right balance between the flexible, adaptive nature of neural networks and the rigid, rule-bound nature of symbolic AI is a key challenge. Overemphasis on one can negate the benefits of the other.
Scalability Issues
Handling Large-Scale Data: While symbolic components improve efficiency and reasoning, they often struggle with the scale at which neural networks operate. Scaling Neurosymbolic AI to handle enterprise-level, big data applications while maintaining the integrity of symbolic reasoning remains a technical hurdle.
Resource Intensiveness: The computational resources required to run integrated systems can be substantial, especially when dealing with complex models and large datasets.
Data Privacy and Security
Vulnerability to Attacks: Like all AI systems, Neurosymbolic AI is susceptible to various types of cyberattacks, including data poisoning and model inversion attacks. The integration of systems potentially exposes new vulnerabilities.
Ensuring Data Privacy: Complying with global data protection regulations (like GDPR) while training sophisticated models that combine personal data and rule-based processing is an ongoing challenge.
Theoretical Foundations
Underdeveloped Theoretical Models: The theoretical underpinnings of combining neural and symbolic AI are not fully developed. Research is needed to better understand the dynamics of this integration, particularly how changes in one system affect the other.
Lack of Standardized Benchmarks: There is a dearth of benchmarks for evaluating the performance of Neurosymbolic AI systems, which complicates the development and comparison of new approaches.
Ethical and Social Implications
Bias and Fairness: Ensuring that Neurosymbolic AI systems do not perpetuate or exacerbate biases present in data or decision-making rules is critical. This includes addressing fairness in automated decisions, especially in sensitive applications like hiring or law enforcement.
Transparency and Accountability: While symbolic AI enhances interpretability, the overall system’s decision-making process must be transparent and explainable to users, especially when used in critical applications. Accountability in cases of errors or failures also needs clear delineation.
The Future of Neurosymbolic AI
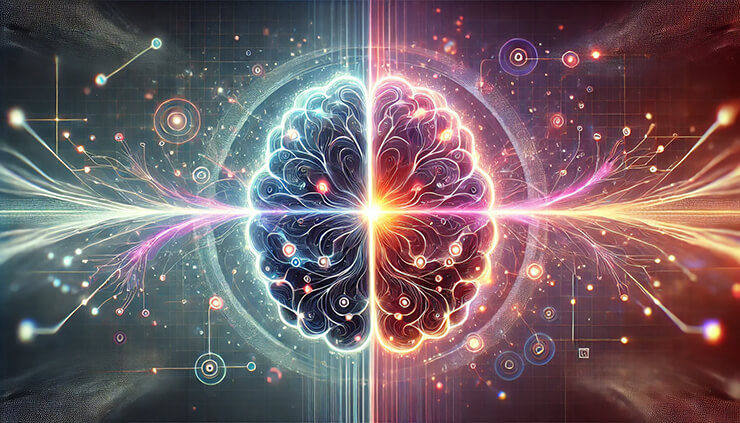
As Neurosymbolic AI continues to evolve, its impact on both technology and society is expected to grow significantly. This section will explore the potential future developments in this field and consider the broader implications of its advancement.
Technological Advancements
Enhanced Algorithms: Future developments are likely to include more sophisticated algorithms that better integrate neural and symbolic processes. This will enhance the system's efficiency and effectiveness, enabling it to handle more complex tasks and larger datasets with greater precision.
Improved Integration Techniques: As researchers gain a deeper understanding of how to balance the strengths and weaknesses of neural networks and symbolic AI, we can expect more seamless and robust integration techniques. These advancements will facilitate broader applications of Neurosymbolic AI across different sectors.
Automation of More Complex Tasks: With improvements in technology, Neurosymbolic AI could automate tasks that currently require significant human judgment and intuition, such as advanced legal reasoning, complex financial planning, and personalized medicine.
Industry Adoption
Broader Industry Penetration: As the technology matures, more industries will likely adopt Neurosymbolic AI to solve specific problems that are currently intractable with existing AI technologies. This could lead to significant shifts in how businesses operate, particularly in data-intensive sectors like healthcare, finance, and law.
Creation of New Job Roles: The rise of Neurosymbolic AI will create new job roles focused on developing, managing, and overseeing these advanced systems. Professionals will need a blend of skills in data science, artificial intelligence, and domain-specific knowledge.
Societal Impact
Ethical AI Development: Neurosymbolic AI could lead to more ethical AI systems due to its inherent capability for transparency and rule-based reasoning. This could help address societal concerns about the opaqueness of AI decision-making processes.
Enhancing Global Accessibility: By reducing the reliance on large datasets and enabling more efficient learning processes, Neurosymbolic AI could make advanced AI technologies more accessible to organizations and countries with limited resources.
Challenges to Overcome
Addressing Bias and Fairness: Ongoing efforts will be needed to ensure that Neurosymbolic AI systems are free from bias and can make fair decisions, especially as they are deployed in more critical areas.
Regulatory and Governance Frameworks: As with any advancing technology, creating appropriate regulatory and governance frameworks will be crucial to manage the deployment of Neurosymbolic AI, ensuring it is used safely and responsibly.
Conclusion
Neurosymbolic AI represents a pioneering approach in the realm of artificial intelligence, blending the intuitive power of neural networks with the logical rigor of symbolic AI to forge systems that are not only smarter but also more understandable and ethical. As we have explored, this integration promises to enhance decision-making, increase data efficiency, and provide a level of transparency that is crucial for trust and accountability in AI applications.
Recap of Key Points
Robust Integration: Neurosymbolic AI combines the pattern recognition strengths of neural networks with the deductive reasoning capabilities of symbolic AI to handle complex tasks that neither could manage alone effectively.
Broad Applications: From improving natural language processing to advancing robotics and streamlining complex decision-making systems in healthcare and finance, the applications of Neurosymbolic AI are broad and impactful.
Addressing Challenges: While the path forward includes significant technical and ethical challenges, ongoing research and development are poised to overcome these hurdles, enhancing the technology's robustness and applicability.
Looking Forward
The future of Neurosymbolic AI is not just about technological advancement but also about its potential to democratize AI, making it more accessible and beneficial across diverse sectors globally. As this technology continues to evolve, it will likely play a critical role in shaping the next generation of AI systems, making them more adaptable, ethical, and capable of genuine understanding and reasoning.